Beyond the hype: the real value of AI today
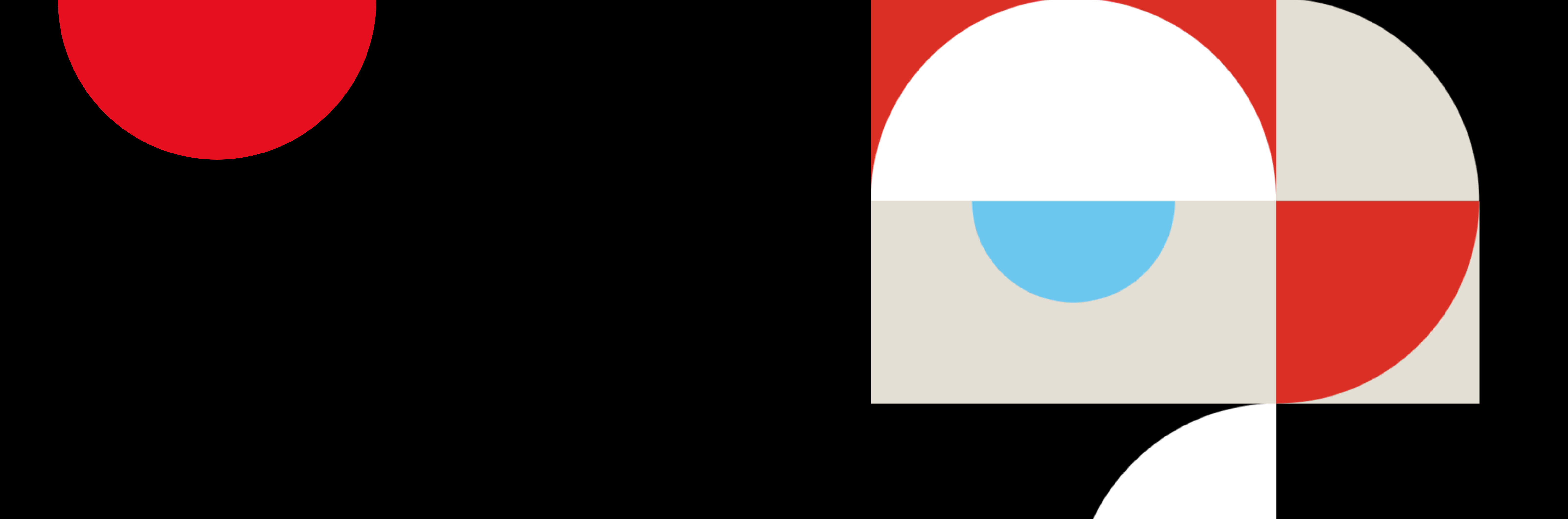
It’s May 8, 2018. At Google’s I/O conference, Google CEO Sundar Pichai is demonstrating Duplex, a new AI feature in Google Assistant. He tells his phone that he wants to set up a hair appointment that fits his schedule. Google Duplex then dials the salon and conducts a voice conversation with the person on the other end. The audience is baffled by how realistic the exchange is: with pauses, breaks, and the casual “mhm”—all the hallmarks of informal human interaction. Duplex doesn't sound like a computer but like a real person making a phone call for an appointment. It’s small and it’s specific, but it made us think: ”Did Google just pass the Turing test?”
Hindsight is 2020
Two and a half years later, it is clear that Google Duplex didn’t turn out to be the next big leap in automated personal assistance but rather an early beta feature that is still being tested in a very controlled and limited environment, with humans in the loop at every step of the way. Does this mean AI is still a long way from delivering deployable and impactful features? No, sir.
Similar to the early maturity stages of other technologies, AI has become the victim of ’hype features’ such as Google Duplex, which are announced as THE. NEXT. BIG. THING. Enthused by these hypes, many organizations realize they should start investing in AI and then struggle to find business value.
In order to generate value from AI today, we need to look beyond the hype. The current strength of AI technology is its ability to recognize patterns in data, and that’s exactly how it is being used across many industries today: AI systems are helping pathologists to analyze tissue samples and make more accurate diagnoses, while Bytedance’s TikTok uses AI to help content creators create viral videos by getting them to the right viewer with an unparallelled recommendation system. While we’re writing this article, Google docs is auto-completing our sentences, increasing our productivity in small increments.
So, what’s the problem?
The struggle to generate business value using AI is not due to a lack of trying. When we talk to business leaders about this challenge, they tell us they’re investing significant time and resources in AI proof of concept (POC) initiatives. While these projects are a great way to get people excited about the technology, they are often centered around the technology itself, with AI becoming a hammer searching for nails to hit. Not surprisingly, these POCs rarely make it into production and fail to accrue business value. A 2019 study from Mindtree—surveying 650 global IT leaders—confirms that only 31% of AI projects deliver a return on investment.
AI POCs are often driven by teams that operate from the organization’s ‘sandbox’ or ‘innovation lab’. Even when successful, it often proves to be challenging or even impossible to take the POC to the next level and implement it in the organization’s real-world complexity. As it becomes impossible to make a business case for AI, projects are shelved and forgotten. This brings us to the million-dollar question: how do you realize the full potential of AI today, and even more so in the future?
Creating business value with AI
The hype surrounding AI has caused many organizations to wrongfully believe that the technology is some kind of ‘silver bullet solution’ that should play by its own rules.
By falling in love with great solutions, we lose track of what really matters: the problems we wanted them to solve.
Focus on the problem, not the solution
Similar to any other technology, the purpose of AI is to address a specific challenge. When identifying potential solutions for any given need, adopting a technology-agnostic view is critical. This means we need to consistently assess whether or not a specific use case would benefit from AI, and always consider other (non-)technological options as well. This mindset allows us to shift from considering AI as a definite solution to assessing whether we’re addressing real needs to begin with.
Identifying problems that can be solved with AI hasn’t been easy. Focusing on ‘groundbreaking AI solutions’ announced by AI frontrunners will give you a preview of what’s to come, but these products should be considered prototypes. They mark technical milestones, with some of these components trickling down into production in the coming years.
Focusing on the problem: explained
You can’t focus on the problem without understanding the challenges AI can solve.
Today, AI’s value lies in three distinctive use cases.
It can be used to automate human tasks. This is especially beneficial for tasks that are considered unsafe, tedious, or inefficient for humans to perform. Typical examples of these are smart email categorization (Gmail) or even self-driving mining trucks (Rio Tinto).
Tasks that will benefit from augmentation, on the other hand, are tasks where AI complements human abilities, providing your business with a ‘superpower’ to unleash improvements in cost, performance, and quality. Examples are using AI to detect fraud-related signals in banking transaction data (Barclays), to track and analyze price elasticity in order to reduce energy costs and negative price variance for users (Honeywell), or to accelerate new vehicle development (Nissan).
A final use case for businesses is to productize AI and offer AI-powered products or services to end-users. In order to successfully productize, AI and other technologies must be used to address real user needs. Philips’ SmartSleep DeepSleep Headband, for example, applies deep learning to understand users’ sleep cycles and helps them improve their sleep quality by intervening with customized audio tones that extend the duration of deep sleep.
Democratize AI knowledge
We are seeing a shift in the accessibility of AI tools that will help organizations to derive business value from AI. Think easy-to-use APIs that can be readily implemented without a specific AI background, making developers less reliant on data scientists. The availability of such APIs enables organizations to prototype specific AI use cases much more quickly, without requiring deep in-house AI expertise.
One example is Google’s Vision API, which offers out-of-the-box solutions such as object detection, landmark detection, and optical character recognition, which are able to accurately predict predefined categories of objects.
In addition, the field of AI offers a wide variety of knowledge-sharing opportunities today, tailored to different levels of AI expertise. While not everyone needs to become an AI engineer or data scientist, this knowledge spillover provides people in a wide variety of organizational roles with access to the foundational knowledge behind AI and the related technology stack. A basic level of understanding of these concepts by product owners, salespeople, strategists, process automation engineers (and many other roles), will increase the likelihood that opportunities to solve business challenges with AI will be recognized. And —even more importantly—that they will recognize the challenges AI won’t be able to solve.
Adopt a data strategy
AI is only as good as the data sources used to train the algorithm. Making high-quality datasets accessible to the entire organization in a uniform way will increase the project success rate and will enable you to scale AI initiatives across the organization.
A solid data strategy will not only enable you to train and test models much more quickly—it will also help you deliver results in the long-term. Training a model on a data dump from one of your business units or customers is one thing, but getting this AI solution into production by exposing new data examples from other business units or users to this model and returning predictions is a far bigger challenge. Getting a data strategy in place, focused on evolving from vertical data silos to a horizontal structure where data is accessible to the entire organization in a uniform way, will simplify the deployment of AI solutions to production and will reduce friction to start AI projects in the first place.
In conclusion
With AI, business leaders should look beyond the hype and identify the customer or business needs AI can solve. While it may be easier to make a business case for automating certain tedious tasks performed by humans today, they should also set out to identify opportunities where AI can be an enabler for new products and services.
The democratization of AI means that AI is ready to become a key component of your organizational strategy, design, and technology toolkit. By releasing AI engineers from the innovation lab and bringing data and AI into the core of your organization—from strategy to operations—you will stop limiting AI initiatives to ‘just another Proof of Concept’, and start embracing the technology as one of your strategic options to address core business challenges.